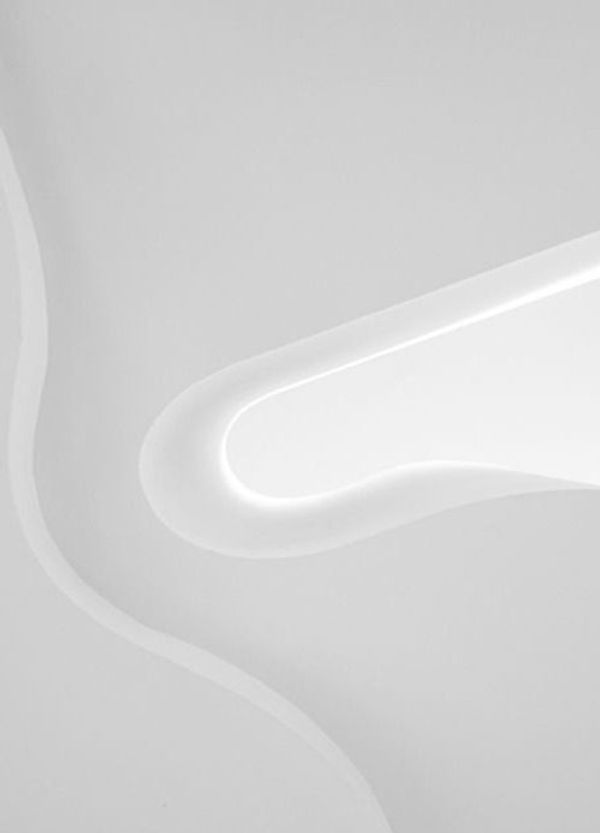
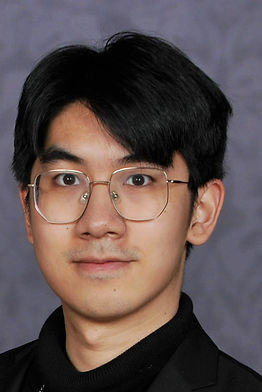
Yunjia Xia
Yunjia Xia is a PhD student at HUB of Intelligent Neuro-Engineering (HUBIN) of the Division of Surgery and Interventional Science at the University College London (UCL). He is also a member of DOT-HUB at the UCL. His project is mainly focused on AI-supported wearable brain imaging and brain computer interface. Prior to his PhD study, he received his MRes and BEng degrees from the UCL-University of Cambridge, United Kingdom, and Beihang University, China, respectively.
Research Interests
-
FPGA Development and Application in Wearable and Interventional Medical Technologies
-
AI Hardware Optimization for Enhanced Functional Brain Imaging
-
Brain-Computer Interface (BCI) for Rehabilitation and Healthcare Monitoring
-
Advanced Electronics Integration in Medical Devices for Improved Healthcare Solutions
Publications
-
Xia, Y. et al. (2024). An FPGA-based, multi-channel, real-time, motion artifact detection technique for fNIRS/DOT systems. IEEE International Symposium on Circuits and Systems (ISCAS), 32(4), 763-773.
-
Ercan, R., Xia, Y. et al. (2024). A real-time machine learning module for motion artifact detection in fNIRS. IEEE International Symposium on Circuits and Systems (ISCAS)
-
Ercan, R., Xia, Y. et al. (2024). An Ultralow-Power Real-Time Machine Learning Based fNIRS Motion Artifacts Detection. IEEE Transactions on Very Large Scale Integration (VLSI) Systems.
-
Xia, Y. et al. (2023). A low-cost, smartphone-based instant 3D scanning system for infant fNIRS applications. Neurophotonics, 10(4):046601.
-
Chen, J., Xia, Y. et al. (2023). fNIRS-EEG brain computer interface for rehabilitation. Bioeigneering, 10(12).
-
Zhou, X., Xia, Y. et al. (2023). Review of recent advances in frequency-domain near-infrared spectroscopy technologies. Biomedical Optics Express, 14(7), 3234-3258.
-
Xia, Y. et al. (2023). A remote-control, smartphone-based automatic 3D scanning system for fNIRS/DOT applications. Optica Biophotonics Congress: Optics in the Life Sciences.
-
Zhao, Y., Xia, Y. et al. (2023). Edge acceleration for machine learning-based motion artifact detection on fNIRS dataset. The 11th IWOCL.